
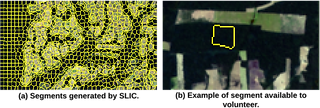
Rainforests play an important role in the global ecosystem. However, significant regions of them are facing deforestation and degradation due to several reasons. Diverse government and private initiatives were created to monitor and alert for deforestation increases from remote sensing images, using different ways to deal with the notable amount of generated data. Citizen Science projects can also be used to reach the same goal. Citizen Science consists of scientific research involving nonprofessional volunteers for analyzing, collecting data, and using their computational resources to outcome advancements in science and to increase the public's understanding of problems in specific knowledge areas...

This paper focuses on one of the most fascinating and successful, but challenging generative models in the literature: the Generative Adversarial Networks (GAN). Recently, GAN has attracted much attention by the scientific community and the entertainment industry due to its effectiveness in generating complex and high-dimension data, which makes it a superior model for producing new samples, compared with other types of generative models. The traditional GAN (referred to as the Vanilla GAN) is composed of two neural networks, a generator and a discriminator, which are modeled using a minimax optimization. The generator creates samples to fool the discriminator that in turn tries to distinguish between the original and created samples...

Computer-aided Diagnosis systems (CAD) have attracted the attention of researchers around the world due to the great success achieved in helping healthcare specialists in the interpretation, analysis, identification and treatment of numerous existing diseases. These systems can be computational processes, models or tools which they assist the specialists through the use of database techniques, image processing, computer vision and artificial intelligence applied to different data modalities such as images, signals, videos and textual documents.
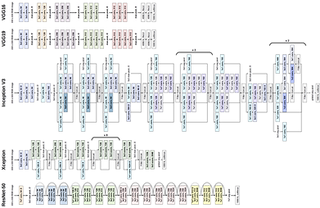
Towards the Robustness in Deep Learning Architectures
Deep learning architectures, in particular the convolutional neural networks (CNNs) are responsible for recent research advances in computational vision and machine learning areas. Due to the fact these networks have achieved excellent results in different application domains. In 2014, a Google research group found that several machine learning models were vulnerable to adversarial examples. The addition of imperceptible noise in images was enough to fool any trained machine learning model. This fact has leveraged a new research field, adversarial pattern recognition, which aims the creation of robust learning models to data distribution different from used in the training process (adversarial examples).
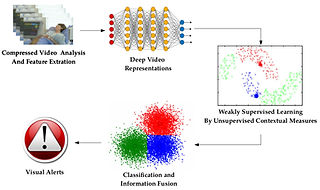
Several machine learning techniques have relied on large labeled data sets to construct predictive models and solving supervised learning tasks. The use of deep learning techniques can be highlighted, since it have been broadly and successfully used in various domains. On the other hand, in many circumstances, the labeled sets are unavailable or insufficient to train effective supervised models. Such scenarios have been mainly addressed by unsupervised learning techniques, which consider the unlabeled data to learn about its structure...
7

Fruit flies are of huge biological and economic importance for the farming of different countries in the World, especially for Brazil. Brazil is the third largest fruit producer in the world with 44 million tons in 2016. The direct and indirect losses caused by fruit flies can exceed USD 2 billion, putting these pests as one of the biggest problems of the world agriculture. In Brazil, it is estimated that the economic losses directly related to production, the cost of pest control and in the loss of export markets, are between USD 120 and 200 million/year...
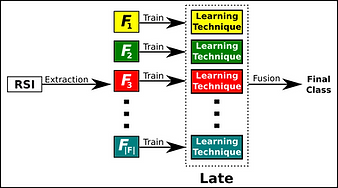
Multiple Classifier System, Stacking, and Ensemble
Machine learning-based solutions have been applied to several complex applications (e.g., data categorization in search, biometric recognition, and document indexing through visual content, and object recognition). Since there is no a silver bullet that achieves good results in any kind of application (“No Free Lunch” theorems), a good alternative might be combining different learning methods to take advantage of complementary information provide from those methods and improve classification results.
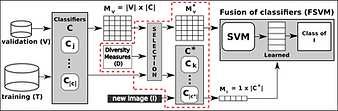
Since there is a huge amount of learning techniques proposed in the literature to solve different application domain and the use of all techniques might become an impracticable task. Ensemble Selection and Pruning approaches aim to reduce the amount of learning technique used on the fusion process maintaining similar effectiveness results than using whole set.
Ensemble Selection and Pruning
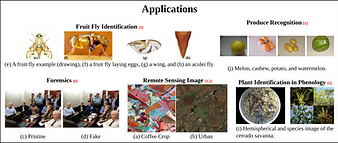
Data Mining and Pattern Recognition in eScience
Data mining (DM) is an interdisciplinary research area composed of different other areas such as artificial intelligence, data base, multimedia data processing, mathematics, statistical, and data visualization. DM area aims extract new, useful, and understandable knowledge from a databases. Pattern recognition techniques have been applied for discovering knowledge in several kind of applications in eScience domain (Pests Recognition, Produce Classification, Forensics Recognition, Crop Recognition, and Vegetation Monitoring).