top of page
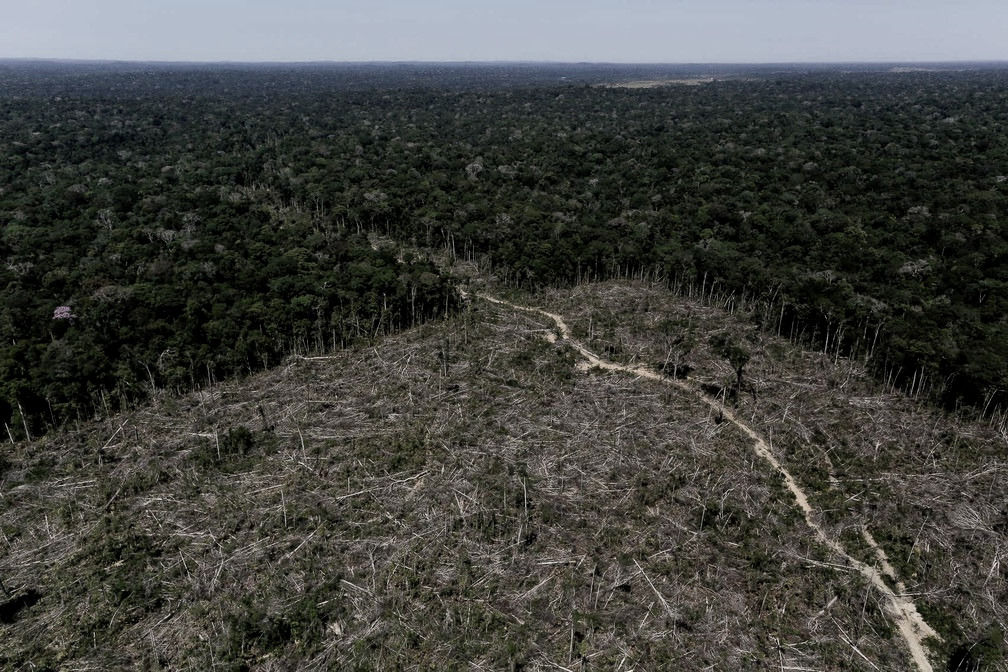
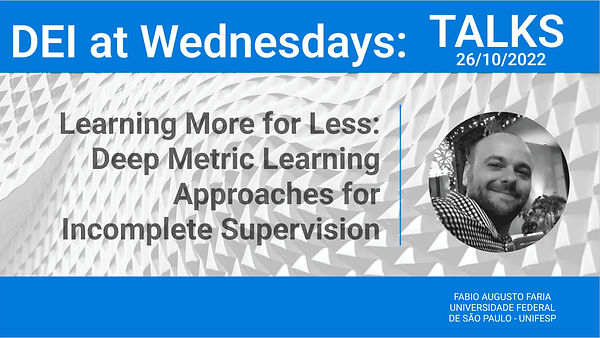
Deep learning architectures (DL) have achieved promising results in different areas (e.g., medicine, agriculture, and security). However, using those powerful techniques in many real applications becomes challenging due to the large labeled collections required during training. Several works have pursued solutions to overcome it by proposing strategies that can learn more for less, e.g., supervised (transfer and few-shot learning), weakly (inexact, inaccurate, and incomplete supervision) and semi-supervised (label propagation and pseudo-labeling) learning approaches. As these approaches do not usually address memorization and sensitivity to adversarial examples of the DL, this paper presents new deep metric learning approaches combined with Mixup technique for incomplete-supervision scenarios. We show that some state-of-the-art approaches in deep metric learning might not work well in such scenarios. Moreover, the proposed approaches outperform most of them in different and well-known datasets. Finally, we employ our approaches for complete scenarios (image classification tasks), achieving excellent classification results compared to the original version approaches in the literature.
Abstract

Oficial URL on Instituto Superior Técnico (IST) em Lisboa - Link
Presentation - Link
Published Paper
-
Luiz H. Buris*; Daniel C. G. Pedronette; Joao P. Papa; Jurandy Almeida; Gustavo Carneiro; Fabio A. Faria*. Mixup-based Deep Metric Learning Approaches for Incomplete Supervision. International Conference on Image Processing (ICIP), 2022. [link]
*L.H.Burisand F.A.Faria contributed equally to this work (co-first authorship).
Acknowledge
The authors are grateful to the São Paulo Research Foundation (FAPESP) and Microsoft Research grants 2021/01870-5 and 2018/23908-1, FAPESP grants 2014/12236-1, 2017/25908-6, and 2019/07665-4, to the National Council for Scientific and Technological Development (CNPq) grants 308529/2021-9 and 314868/2020-8, and to NVIDIA for donating a TitanV GPU used in the experiments.
bottom of page